How Medical Treatment Algorithms Are Shaping the Healthcare Industry
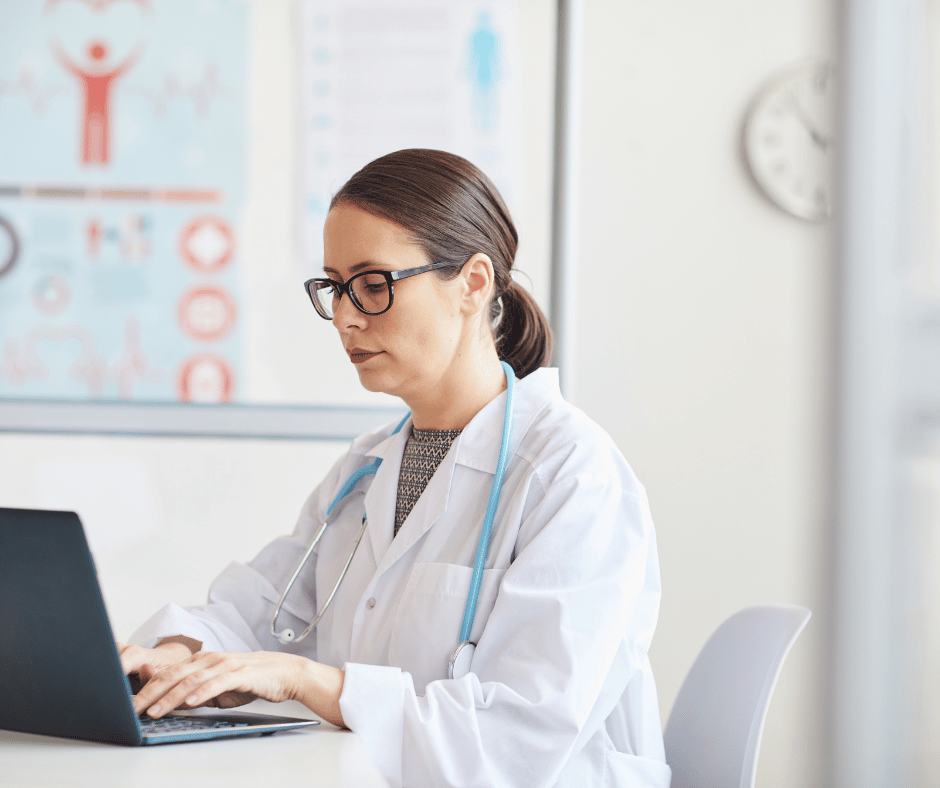
The healthcare industry is rapidly evolving with technology alongside it. With more than 80% of hospitals and medical practices implementing Artificial Intelligence (AI), the importance of constructing and monitoring these growing technologies is crucial. This is also why medical treatment algorithms have recently become such important factors in closing patient care gaps. By creating algorithms in healthcare that standardize the way patients are treated and bolster the predictive capabilities of big data, healthcare professionals will be able to treat more patients in less time. Let’s look further into medical treatment algorithms, what we should consider when implementing them, and how they will be beneficial to the healthcare industry as a whole.
What Are Medical Treatment Algorithms?
Medical treatment algorithms are mathematical sequences or sets of rules that help solve problems or inform decisions in patient care. Many different health algorithms are used for various purposes. Some algorithms use tables, nomograms, or flowcharts to make decisions or treatment plans for patients. Others use AI, Machine Learning (ML), and Natural Language Processing (NLP) to predict the likelihood of patient health. This substantial amount of data is known as big data and is collected primarily from healthcare providers’ Electronic Health Records (EHR).
As the volume of medical data increases, the sets of rules within a medical algorithm can guide users on how to treat a patient or predict what conditions the patient may be susceptible to. In general, medical treatment algorithms work in three steps: inputting data, processing data, and making recommendations based on the aforementioned data. Sometimes this can be simple, like in a flowchart. In a flowchart, the inputted data comes from observations from a physician. It’s then processed by administering the observations through each step of the flowchart. Based on the processing of the flowchart, a recommendation is made. A healthcare provider can either take action by accepting that recommendation or making one of their own based on separate observations.
With more data, however, medical treatment algorithms can become more complicated. While the process is somewhat similar, instead of using observations, Machine Learning algorithms use statistics and population data to find patterns within data. Over time, these systems learn from their data and improve upon the recommendations they make in the future.
Advantages of Using Medical Treatment Algorithms
Increased Accuracy in Diagnosis and Treatment
The main benefit of combining medical treatment, algorithms, and technology is the increased accuracy in diagnosing and taking care of patients. To make the correct diagnosis, it is integral to use an algorithm that provides a basis by which doctors can easily and accurately identify the symptoms and conditions of a patient. When these medical algorithms are used by care providers, it standardizes the way patients are cared for, minimizing differences and inequality of care overall. With more complicated data sets, which are becoming increasingly common in the healthcare industry, having a treatment algorithm that can analyze complex data, predict the likelihood of ailments, and personalize medical care per patient while continually improving upon itself will increase the accuracy of diagnosing and treating patients as time goes on.
In addition, a study done by the U.S. Government Accountability Office (GAO) found that there are technologies available in the U.S. that are detecting diseases earlier and delivering more consistent analysis of medical data. This has increased patients’ access to care, particularly for underserved populations afflicted with certain cancers, diabetic retinopathy, Alzheimer’s disease, heart disease, and COVID-19. What medical professionals must keep in mind, however, is when low-quality data is inputted into medical treatment algorithms, it may lead to inconsistencies or poor performance.
Improved Efficiency and Cost-Effectiveness in Healthcare Delivery
Beyond just the increased accuracy of medical treatment algorithms, there are also efficiency and cost-effectiveness factors to keep in mind. Think about it like this: with more treatment algorithms that can streamline diagnoses, doctors can avoid time-consuming testing and data analysis that commonly prevent them from giving patients their best treatment possible. While that helps in real-time, health algorithms can help increase efficiency and cost-effectiveness in the future by identifying patients with certain traits who are at a higher risk of developing conditions. If doctors are alerted to these patients sooner, they can first work together to prevent those conditions from possibly developing, and if they do develop, they can treat them earlier, reducing hospital readmissions and resources expended on extended care.
Limitations and Ethical Concerns
One main concern as the use and complexity of medical algorithms continue to progress is their overuse, misuse, and rationalization of them. Rather than inherently trusting medical algorithms, especially those which utilize AI, there should always be manual oversight by a physician. For the time being, advanced health algorithms are not infallible, so being sure to use as much data as possible when collaborating with AI, rather than conceding, will be important going forward.
Beyond the technical limitations of algorithms in healthcare, there are still certain ethical considerations that must be considered, especially when it comes to underserved communities. Often, medical algorithms are constructed without considering patient populations as a whole. For example, in a 2019 study, it was concluded Black patients received a lack in quality care compared to non-Black patients according to a risk-prediction algorithm that determined a patient’s need for care based on previous healthcare spending.
While the algorithm’s purpose may have been positive, it unintentionally disqualified Black patients from receiving extra care due to their lack of spending on healthcare services. Where the controversy lies is that this limited spending was not due to a lack of need from Black patients: it was due to a lack of resources that prevented these patients from seeking medical care. This thereby diminished the amount spent and suppressed Black patients from receiving the extra treatment they needed.
These limitations and ethical concerns must be addressed as the use of medical treatment algorithms progresses forward and providers continue to try to close patient care gaps. However, whether the concerns outweigh the benefits will be a prominent point of discussion amongst medical professionals and researchers in the field.
The Future of Medical Treatment Algorithms
Medical treatment algorithms are being perfected constantly. However, there are ways to close patient care gaps without relying completely on medical algorithms. One of those is by preventing blindness in adults with diabetes.
Diabetes is the leading cause of blindness in adults; however, it is preventable. With the IRIS Solution, both stationary and mobile healthcare providers can close patient care gaps by offering teleretinal screening exams that allow for the detection of diabetic retinopathy with 95% accuracy in just a couple of hours. To learn more about the impact of IRIS, contact us, or read more from our case studies to see the IRIS Solution in action.
Frequently Asked Questions
How Are Algorithms Used in Healthcare?
Algorithms in healthcare play a crucial role in improving diagnostics, treatment, and patient care. They help analyze large amounts of data quickly, identifying patterns and making predictions that allow healthcare professionals to make more informed decisions. Medical treatment algorithms are transforming healthcare, driving precision medicine, and improving patient outcomes.
What Is the Current Treatment Algorithm?
Current treatment algorithms in healthcare can range from visual aids such as tables, nomograms, and flowcharts to complex models powered by Artificial Intelligence. These tools facilitate consistent and efficient clinical decision-making, ensuring that patient care is both personalized and accurate.
What Is a Clinical Algorithm?
A clinical algorithm is a structured methodology used in healthcare to guide decision-making processes. Often represented visually, it is a series of steps that aid medical professionals in diagnosing and managing a patient’s condition. As healthcare continues to digitize, the integration of technology into these algorithms, will revolutionize predictive medicine and enable early intervention in diseases like diabetic retinopathy.
What Are the Different Types of Medical Algorithms?
Medical algorithms fall into three main categories: diagnostic, predictive, and Machine Learning. Diagnostic algorithms help confirm diseases, predictive algorithms estimate disease likelihood, and Machine Learning algorithms use data to detect patterns for early diagnosis and treatment strategies.
SM# 142, Rev A
Get started with IRIS today.
Want to know if IRIS is right for you? Schedule a one-on-one consultation with our team. We’re here to help.